Hearing Aids and Machine Learning: Improving Sound Quality
Last Updated on 18/04/2025 by Admin
Unlocking the Power of Machine Learning in Hearing Aids
Machine learning has rapidly become a groundbreaking element in the realm of audiology, profoundly transforming the functionality of hearing aids to better meet the unique needs of individual users. By leveraging advanced algorithms that process and analyze extensive data sets, modern hearing aids equipped with machine learning capabilities demonstrate enhanced efficiency in sound processing, customization of user experiences, and the delivery of a clearer auditory environment. This remarkable evolution in hearing technology not only enriches the user experience but also fosters greater independence and improved communication skills, effectively addressing a diverse spectrum of hearing challenges that individuals face around the world.
Understanding the Fundamentals of Machine Learning
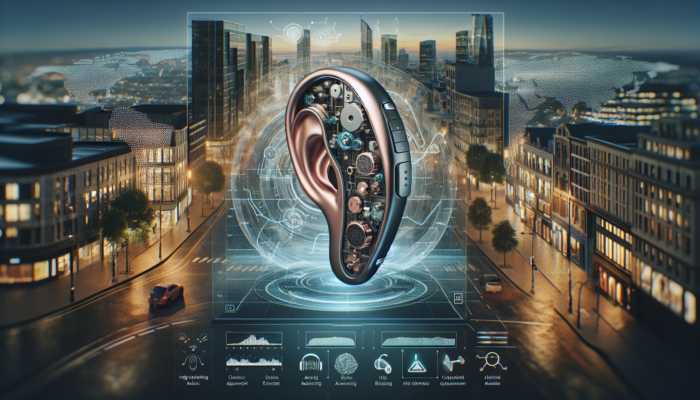
Machine learning constitutes a vital segment of artificial intelligence that focuses on creating algorithms capable of learning from data and making informed predictions. Rather than being explicitly programmed for specific tasks, these algorithms evolve and enhance their functionality as they encounter more data inputs. This self-optimizing capability is invaluable in the context of hearing aids, where the intricacies of sound processing are influenced by multiple factors, including background noise, speech patterns, and individual hearing profiles. Machine learning models in hearing aids are meticulously trained to recognize various sound environments, allowing them to optimize audio output in real-time, thereby significantly improving the user experience.
As users interact with their hearing aids, the devices continuously gather data regarding their listening preferences and the specific environmental conditions they encounter. This collected information is analyzed to allow hearing aids to personalize sound output for each unique context. The integration of machine learning with hearing aids not only enhances sound clarity but also enables users to engage more meaningfully in social situations, demonstrating how the application of machine learning in hearing aids is revolutionizing the field of hearing health.
Exploring the Advantages of Machine Learning in Hearing Aids
The incorporation of machine learning into hearing aids provides numerous advantages that significantly elevate the user experience. One of the primary benefits is the substantial improvement in sound processing capabilities. Traditional hearing aids often faced challenges in sifting through background noise, making conversations difficult in crowded settings. However, with the implementation of machine learning algorithms, these devices can now adeptly differentiate between noise and speech. This advanced capability allows users to enjoy clearer conversations, even in bustling environments like restaurants or busy streets, fundamentally enhancing their auditory experiences.
In addition, machine learning facilitates personalized adaptability in hearing aids. Every user possesses distinct hearing needs and preferences, which can vary across different environments. Machine learning algorithms meticulously analyze user behavior and preferences over time, crafting bespoke sound profiles that resonate with each individual. This personalized adaptability ensures that hearing aids are tailored auditory companions, rather than mere one-size-fits-all devices, evolving alongside the user’s needs and enhancing their overall satisfaction.
Another significant advantage of incorporating machine learning into hearing aids is the reduction of the trial-and-error approach often associated with fitting and adjusting these devices. Traditionally, users relied heavily on professional adjustments, which could be cumbersome and time-consuming. Hearing aids equipped with machine learning capabilities can autonomously adjust settings based on real-time data, significantly enhancing user comfort and satisfaction without the need for constant professional intervention.
Tracing the Evolution of Machine Learning in Hearing Technology
The integration of machine learning into hearing technology marks a remarkable journey of innovation within the field of audiology. Initially, hearing aids primarily focused on amplification, paying little attention to the complexities of sound processing. However, as technology evolved, the limitations of conventional methods became increasingly evident, particularly in intricate sound environments. The introduction of digital signal processing brought significant advancements to hearing aids, but the real breakthrough was achieved with the advent of machine learning technologies.
Early applications of machine learning in hearing aids were relatively basic, concentrating on fundamental sound classification tasks. Nevertheless, as algorithms gained sophistication and the availability of data expanded, hearing aids began to offer advanced features like automatic noise reduction and adaptive microphones. Recognizing the value of user feedback and data analysis, manufacturers started collaborating with researchers in machine learning to enhance the algorithms powering these innovative devices.
Today, machine learning stands at the forefront of hearing aid technology, with ongoing research exploring advanced applications such as real-time speech enhancement and context-aware adjustments. The historical transition from simple amplification to intelligent, adaptive devices reflects an increasing understanding of user needs and the technology’s potential to meet those demands effectively.
Current Innovations in Machine Learning Applications for Hearing Aids
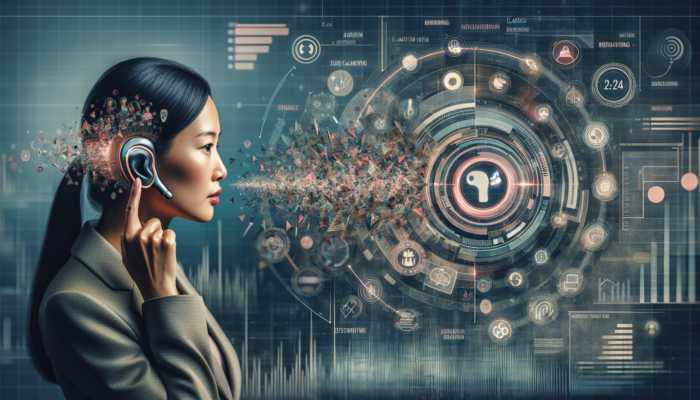
Machine learning is currently leveraged in various groundbreaking applications within hearing aids, significantly boosting their performance and functionality. One of the most notable applications is the advanced noise reduction capabilities. By employing sophisticated algorithms, hearing aids can effectively differentiate between desirable sounds, such as speech, and unwanted background noise, making it easier for users to focus on conversations without being distracted by environmental sounds. This ensures a more enjoyable auditory experience, particularly in challenging settings.
Another critical application of machine learning is in the realm of speech enhancement. Machine learning algorithms analyze audio signals to extract speech more clearly from a cacophony of sounds, facilitating easier conversation tracking even in complex environments. Whether attending a cocktail party or sitting in a bustling café, users can engage in discussions without straining to hear, which is especially beneficial for older adults or individuals with severe hearing loss who may struggle with background noise interference.
Additionally, automatic program selection emerges as another remarkable feature powered by machine learning. Hearing aids can intuitively switch between different sound processing programs based on the user’s environment. For instance, when transitioning from a quiet library to a noisy street, the hearing aid can automatically adjust to optimize sound quality, ensuring that users consistently experience the best auditory output tailored to their surroundings.
Envisioning the Future of Machine Learning in Hearing Technology
The future of machine learning in hearing technology is filled with immense potential. As algorithms continue to evolve, we can anticipate even more advanced personalization features that learn and adapt in real-time, providing users with auditory experiences tailored not only to their hearing needs but also to their emotional and social contexts. This could lead to hearing aids that intuitively adjust based on the user’s mood or the specific dynamics of a conversation, enhancing social connections and communication.
Moreover, the integration of hearing aids with other technologies is looming on the horizon. Imagine a future where hearing aids seamlessly connect with smartphones, smart home devices, and health monitoring tools. This interconnectedness would facilitate comprehensive health tracking that transcends hearing alone, enriching the user experience and providing a holistic view of an individual’s overall well-being.
Artificial intelligence will also play an increasingly significant role in customizing hearing aid settings. As AI technologies advance, we might witness systems that predict user needs even before they are articulated, creating a truly intuitive auditory device. The synergy of machine learning and AI not only enhances the functionality of hearing aids but also positions them as essential tools for improving quality of life, fostering social engagement, and managing overall health.
Enhancing Sound Processing and Quality
Sound processing and enhancement lie at the core of contemporary hearing aid technology, particularly with the infusion of machine learning. The capability of hearing aids to dynamically adapt and refine sound quality in real-time significantly influences user satisfaction and engagement in daily activities. Through advanced algorithms, hearing aids now deliver unparalleled clarity and personalization in sound processing, addressing individual hearing needs more effectively than ever before.
Advanced Noise Reduction Techniques
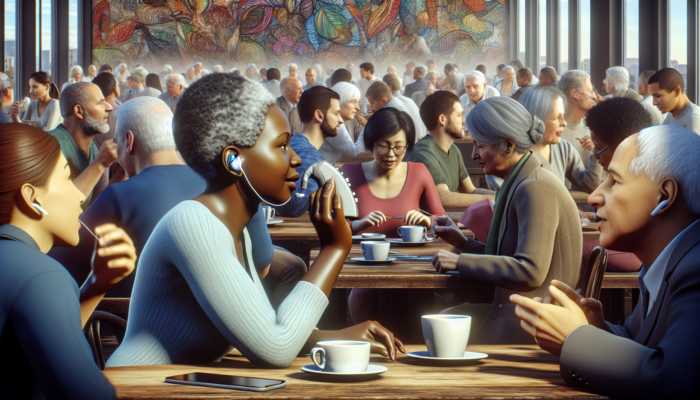
Noise reduction remains one of the most substantial challenges faced by hearing aid users, particularly in crowded or dynamic environments. Traditional hearing aids often struggled to effectively filter out background noise, leading to frustration during conversations. With the emergence of machine learning, however, noise reduction techniques have evolved to become more sophisticated and efficient.
Machine learning algorithms analyze audio input in real-time, distinguishing between different sound sources. By identifying which sounds represent speech and which constitute background noise, these algorithms can apply tailored filters that minimize distractions. For example, during a conversation in a bustling café, the hearing aid can amplify the speaker’s voice while dampening the clatter of dishes and the chatter of other patrons. This capability not only enhances clarity but also empowers users to engage more fully in conversations, making social interactions more enjoyable.
The effectiveness of these noise reduction techniques varies based on the sophistication of the algorithms employed. Some models are designed to adapt according to user preferences, learning over time which sounds to prioritize and which to suppress. This continuous learning process fosters a more personalized experience, ensuring that users can hear what matters most to them. The remarkable ability of hearing aids to utilize machine learning for noise reduction exemplifies how technology can positively transform lives, enabling users to navigate their environments with confidence.
Enhancing Speech Clarity Through Innovation
Speech enhancement represents another critical area where machine learning has made a substantial impact on hearing aids. Understanding speech in noisy environments is a universal challenge for individuals with hearing loss. Machine learning algorithms play a vital role in enhancing speech clarity by intelligently analyzing auditory signals to isolate and amplify speech while suppressing background noise.
These algorithms meticulously process sound waves to identify key features of speech, such as pitch and rhythm. By focusing on these characteristics, hearing aids can deliver clearer audio of conversations, allowing users to actively participate in discussions, regardless of the surrounding noise. This feature is especially beneficial in settings like restaurants, where multiple conversations may occur simultaneously, and background music could complicate the auditory landscape.
Recent advancements in deep learning have further enhanced the capabilities of speech enhancement in hearing aids. By leveraging extensive datasets of speech in various conditions, these algorithms can learn to predict and reconstruct speech signals with remarkable accuracy. This not only improves the clarity of conversations but also reduces cognitive load for users, enabling them to focus on engaging rather than straining to decipher speech.
The impact of enhanced speech clarity extends beyond mere convenience; it fosters social inclusion and emotional well-being. Users can engage more confidently in discussions, significantly improving their quality of life and strengthening their relationships with family and friends, showcasing how the use of machine learning in hearing aids can change lives for the better.
Real-Time Feedback Cancellation Techniques
Feedback in hearing aids occurs when sound from the speaker escapes and re-enters the microphone, resulting in an unpleasant whistling sound. This issue has long been a source of frustration for users. However, with advancements in machine learning, hearing aids are now equipped with sophisticated feedback cancellation techniques that greatly enhance sound quality.
Machine learning algorithms can detect feedback in real-time by analyzing audio signals. Upon identifying feedback, the system can implement corrective measures, such as adjusting gain levels or modifying sound frequencies to eliminate the feedback loop. This real-time processing ensures that users experience a seamless auditory environment devoid of the annoying interruptions caused by feedback.
Moreover, advanced feedback cancellation systems are capable of learning from user experiences. By gathering data on when and where feedback occurs, these systems can refine their response strategies, becoming more effective over time. This adaptability is crucial, as different environments and sound sources can influence the likelihood of feedback occurrence.
The benefits of effective feedback cancellation are profound. Users can enjoy more stable sound quality, facilitating uninterrupted conversations and a more pleasant listening experience overall. By mitigating the impact of feedback, machine learning has not only improved hearing aid functionality but also contributed to a more enjoyable and engaging auditory experience for users.
Personalization Enhancing User Experience
Personalization serves as a fundamental pillar of modern hearing aid technology, particularly through the application of machine learning. Individuals with hearing loss encounter a wide array of auditory challenges, and the capacity to customize hearing aids to address these unique needs is essential for enhancing user experience. Machine learning plays an integral role in developing personalized solutions that optimize the listening experience for each user.
Creating Custom Sound Profiles for Individual Needs
Custom sound profiles signify a major advancement in hearing aid technology, empowering users to achieve a tailored auditory experience. Traditional hearing aids often relied on generic settings, which could leave users dissatisfied with their hearing experience. However, the integration of machine learning allows hearing aids to create personalized sound profiles based on individual preferences and hearing patterns.
Machine learning algorithms analyze data collected from user interactions, including responses to various sound environments and specific listening preferences. By leveraging this data, hearing aids can generate customized sound profiles that adapt to different listening situations, whether in quiet settings, bustling cafes, or outdoor activities. This tailored approach ensures that users experience optimal sound quality and clarity, significantly enhancing their overall satisfaction with the device.
The development of custom sound profiles not only enriches the auditory experience but also empowers users to take control of their hearing preferences. Many hearing aids equipped with machine learning come with user-friendly interfaces that enable individuals to adjust settings according to their needs easily. This level of personalization fosters a sense of ownership and comfort, encouraging users to engage more actively in their social interactions and communication.
As machine learning technology continues to evolve, users can anticipate even more sophisticated algorithms that refine custom sound profiles over time. The adaptability of these systems will facilitate continuous improvement, ensuring that users can enjoy an auditory experience that evolves alongside their changing preferences and environments.
Embracing Adaptive Learning Capabilities
Adaptive learning represents a remarkable feature of machine learning in hearing aids, allowing devices to automatically adjust to different listening environments. Users frequently encounter varied soundscapes in their daily lives, ranging from tranquil libraries to lively gatherings, and the ability of hearing aids to adapt to these changing conditions is crucial for a positive auditory experience.
Machine learning algorithms continuously analyze sound data from the user’s environment, identifying the specific characteristics of different listening scenarios. For instance, in a noisy setting, the hearing aid may enhance speech frequencies while diminishing background noise, ensuring that conversations remain clear and intelligible. Conversely, in quieter settings, the device may prioritize natural sounds and ambient noise to create a more balanced auditory experience.
This adaptive learning process not only benefits sound quality but also enhances user comfort and confidence. When users understand that their hearing aids can automatically respond to their surroundings, they are less likely to feel anxious about missing important sounds or struggling to hear. This confidence translates into improved social interactions and a greater sense of belonging, as users can engage more fully in conversations without the burden of manual adjustments.
Moreover, adaptive learning systems can learn from user feedback and preferences over time, continually refining their responsiveness to different environments. This level of personalization guarantees that hearing aids remain effective tools for communication, emphasizing the critical role of how hearing aids utilize machine learning in shaping positive auditory experiences.
Integrating User Feedback for Continuous Improvement
User feedback integration stands as a vital component in the evolution of hearing aids powered by machine learning. The effectiveness of these devices heavily relies on accurate data regarding user preferences and experiences. By actively incorporating user feedback, manufacturers can optimize hearing aid performance and ensure that users feel heard and understood in their auditory needs.
Hearing aids equipped with machine learning often feature user-friendly applications that allow individuals to provide real-time feedback on their listening experiences. This feedback can include preferences related to volume levels, sound clarity, and comfort in various environments. The machine learning algorithms then analyze this feedback, identifying trends and patterns that can inform future adjustments and enhancements to the device’s performance.
The process of integrating user feedback fosters a sense of collaboration between users and manufacturers. It empowers individuals to take an active role in shaping their auditory experiences, ensuring that their unique hearing needs are adequately met. Furthermore, the continuous learning aspect of machine learning means that hearing aids can evolve alongside users, adapting to their changing preferences and environments over time.
As machine learning technology progresses, we can expect even more refined methods for capturing and analyzing user feedback. Enhanced algorithms may enable hearing aids to predict user preferences based on historical data, further streamlining the personalization process. This integration of user feedback not only enhances the functionality of hearing aids but also nurtures a deeper connection between users and the technology that supports them in their auditory journey.
Personalized Control Interfaces for Enhanced Accessibility
Personalized control interfaces are revolutionizing how users interact with their hearing aids, significantly enhancing accessibility and ease of use. Traditional hearing aids often relied on complex buttons and settings, which could be overwhelming for users, particularly those not well-versed in technology. However, with machine learning integration, control interfaces have become more intuitive and tailored to individual preferences.
Many modern hearing aids offer companion apps that enable users to customize settings conveniently from their smartphones or tablets. These applications typically feature user-friendly interfaces that allow individuals to adjust volume levels, switch between sound profiles, and access additional features, such as noise reduction or speech enhancement, with just a few taps. By empowering users to personalize their auditory experiences according to their specific needs, manufacturers enhance user satisfaction and engagement.
Machine learning algorithms play a crucial role in enhancing these interfaces by learning from user interactions. Over time, the system can identify common adjustments made by users and suggest optimal settings for various environments. For instance, if a user frequently increases volume in social situations, the app could automatically adjust to enhance speech clarity in similar scenarios. This proactive approach not only streamlines the user experience but also fosters a sense of confidence and control.
Additionally, personalized control interfaces can improve accessibility for individuals with varying levels of technical proficiency. By simplifying the process of adjusting settings and offering clear guidance, hearing aids can accommodate diverse user needs, ensuring that everyone can benefit from advancements in hearing technology. The integration of machine learning not only enriches the auditory experience but also signifies a commitment to inclusivity in hearing health.
Context-Aware Adjustments for Optimized Hearing Experiences
Context-aware adjustments represent one of the most exciting advancements in hearing aid technology, driven by machine learning. These adjustments enable hearing aids to automatically modify their settings based on the user’s environment and activities, creating a seamless auditory experience that adapts to ever-changing contexts.
Leveraging sophisticated sensors, hearing aids can detect various situational factors, such as background noise levels, the presence of multiple speakers, or the type of environment—whether it’s a quiet room, a busy street, or a crowded venue. Machine learning algorithms analyze these data points in real-time, allowing the device to make intelligent adjustments that enhance sound quality without requiring any manual input from the user.
For instance, when a user enters a loud environment, such as a concert or a lively family gathering, the hearing aid can automatically increase the amplification of speech frequencies while reducing extraneous noise. Conversely, in quieter settings, the device may prioritize natural ambient sounds, allowing users to remain aware of their surroundings while still benefiting from enhanced hearing capabilities. This level of contextual awareness guarantees that users can enjoy optimal auditory experiences across diverse situations.
The impact of context-aware adjustments transcends mere convenience; it promotes greater engagement and social interaction for users. Individuals no longer have to worry about fiddling with controls or missing important sounds in dynamic environments. By allowing hearing aids to adapt intelligently, users can focus on enjoying their experiences and connecting with others, showcasing the profound influence of how hearing aids leverage machine learning to elevate everyday life.
Comprehensive Data Collection and Analysis
Data collection and analysis serve as foundational elements in optimizing the performance of hearing aids powered by machine learning. The capability to gather and analyze user data enables manufacturers to refine algorithms, enhance device functionality, and ultimately improve user experiences. However, this capability also carries significant responsibilities regarding privacy and security.
Understanding Data Acquisition Methods
Data acquisition methods in hearing aids are critical to comprehending user interactions and environmental conditions. Modern hearing aids are equipped with sensors and microphones that continuously gather data on user experiences, preferences, and the surrounding auditory environment. This information serves as the basis for machine learning algorithms to enhance device performance and personalize user experiences.
The data collected encompasses sound profiles from various environments, user interaction patterns, and feedback on device performance. For example, hearing aids can track how users adjust volume levels in different situations, offering insights into their preferences and enabling the development of custom sound profiles. By analyzing this data, machine learning algorithms can optimize hearing aid settings, ensuring that users receive the best auditory experience possible tailored to their needs.
Data collection occurs continuously as users navigate their daily lives, creating a dynamic process that is vital for refining machine learning algorithms and ensuring that hearing aids remain responsive to users’ needs.
However, it is essential to acknowledge the importance of user consent and transparency in data acquisition. Manufacturers must ensure that users are fully informed about the data collected and how it is utilized. By prioritizing user privacy, manufacturers can build trust and encourage more users to embrace the advantages of data-driven hearing aid technology.
Efficient Data Processing and Storage
Once data is collected, efficient processing and storage are essential for deriving meaningful insights and enhancing hearing aid functionality. Advanced algorithms process the gathered data to identify patterns and trends that can inform adjustments to device performance, which is crucial for enhancing the effectiveness of machine learning models used in hearing aids.
Data processing often involves intricate calculations and analyses, where algorithms evaluate user interactions, sound environments, and preferences. By identifying correlations between user behavior and hearing aid performance, manufacturers can refine their algorithms to create more personalized experiences. For instance, if data reveals that a user consistently adjusts settings in noisy environments, the algorithm can learn to automatically enhance noise reduction in similar situations.
Data storage is equally critical, as manufacturers must ensure that user information is securely stored and compliant with relevant regulations. Protecting user data is vital for maintaining trust and ensuring individuals feel confident in the technology they utilize. By implementing robust security measures, manufacturers can safeguard sensitive information while allowing for meaningful analysis that drives continuous improvement.
Ultimately, effective data processing and storage empower hearing aids to leverage the power of machine learning, creating devices that adapt intelligently to users’ needs and preferences. This capability reflects a commitment to enhancing user experiences and optimizing hearing health outcomes.
Addressing Privacy and Security Concerns
As hearing aids become increasingly advanced and data-driven, privacy and security concerns gain prominence. The collection and processing of user data raise significant ethical questions surrounding confidentiality, consent, and data ownership. Manufacturers bear the responsibility of ensuring that users’ private information is protected while still benefiting from insights gained through data analysis.
Users must be informed about the types of data collected, the rationale behind its collection, and how it will be utilized. Transparency is key to building trust and encouraging users to engage with machine learning-powered hearing aids. Manufacturers should provide clear information regarding data policies, including how data is anonymized and secured.
To address privacy concerns, manufacturers can implement robust encryption and security measures to protect user data from unauthorized access. Additionally, users should retain control over their data, including options to opt-in or opt-out of data collection features. This level of control empowers users to make informed decisions about their engagement with hearing technology while prioritizing their privacy.
Furthermore, regulatory frameworks, such as the General Data Protection Regulation (GDPR) in Europe, outline stringent guidelines for data collection and management. Manufacturers must comply with these regulations to ensure protection and uphold users’ rights. By prioritizing privacy and security, hearing aid manufacturers can foster a sense of confidence and safety among users, encouraging them to embrace the benefits of machine learning technology.
Utilizing Advanced Data Analysis Techniques
Data analysis techniques are pivotal in optimizing the functionality of hearing aids powered by machine learning. By employing advanced statistical methods and machine learning models, manufacturers can extract valuable insights from the collected data, informing device enhancements and improving user experiences.
One common technique used in data analysis is regression analysis, which helps identify relationships among various variables. For instance, regression models can analyze how different environmental factors influence user preferences and hearing aid performance. This analysis allows manufacturers to refine algorithms to better accommodate users’ unique hearing needs.
Additionally, clustering techniques can categorize users based on similar preferences and behaviors. By identifying distinct user segments, manufacturers can develop targeted solutions that address the specific needs of different user groups. This level of personalization enhances user satisfaction by ensuring that hearing aids are tailored to individual preferences and requirements.
Machine learning techniques, such as neural networks, can also be employed to analyze large datasets and identify complex patterns. These algorithms can learn from data over time, continuously improving their performance and adapting to changes in user behavior. The ability to process vast amounts of data enables manufacturers to create more intelligent and responsive hearing aids, ultimately enriching the user experience.
As data analysis techniques continue to evolve, we can expect increasingly sophisticated methodologies that enhance the capabilities of hearing aids. By harnessing the power of data, manufacturers can develop cutting-edge devices that transform the auditory experience for individuals with hearing loss.
Effective Reporting and Visualization of Data Insights
Reporting and visualization of data insights are essential for translating complex analyses into actionable information that informs decision-making for hearing aid manufacturers. By presenting data in clear and intuitive formats, manufacturers can gain valuable insights into user behavior, preferences, and device performance.
Visual dashboards are increasingly utilized in the hearing aid industry to display key metrics and trends. These dashboards enable manufacturers to monitor user interactions and identify areas for improvement. For example, a dashboard might showcase data related to the most common adjustments made by users, shedding light on specific preferences that can inform future enhancements.
Moreover, effective reporting can facilitate collaboration between manufacturers and audiologists. By sharing data insights, audiologists can better understand their clients’ needs and make informed recommendations for device adjustments. This partnership enhances the overall experience for users, ensuring they receive tailored support based on their preferences and behaviors.
Visual tools and reports can also empower users themselves. Many modern hearing aids come with companion apps that provide users with insights into their hearing habits, including usage patterns and the frequency of adjustments. By enabling users to visualize their experiences, manufacturers foster a sense of engagement and ownership over their auditory journey.
Ultimately, effective reporting and visualization of data insights enhance decision-making processes within the hearing aid industry. By translating data into meaningful information, manufacturers can create devices that better serve the needs of users and drive continuous improvement in hearing technology.
Exploring Machine Learning Algorithms in Hearing Aids
The application of machine learning algorithms is essential for the effective functionality of modern hearing aids. These algorithms empower devices to learn from data, adapt to user needs, and optimize performance in real-time. Gaining a deeper understanding of the types of machine learning algorithms employed can provide valuable insights into how hearing aids continually improve the user experience.
Applications of Supervised Learning in Hearing Aids
Supervised learning represents a prominent machine learning approach utilized in hearing aids, where algorithms are trained on labeled datasets to classify and predict sound patterns. In the context of hearing aids, supervised learning proves particularly effective for tasks such as distinguishing between speech and background noise.
By training algorithms on extensive datasets that encompass various sound environments, manufacturers can create models capable of accurately recognizing specific audio patterns. For instance, a supervised learning algorithm can learn to identify speech frequencies and amplify them while suppressing irrelevant sounds. This capability is crucial for ensuring that users can engage in meaningful conversations, even in challenging auditory settings.
Moreover, supervised learning facilitates automatic program selection in hearing aids. By analyzing user interactions and environmental factors, these algorithms can determine the most appropriate settings for different situations, such as restaurants, classrooms, or outdoor gatherings. This level of automation enhances user convenience and ensures that hearing aids deliver optimal performance without requiring manual adjustments.
The efficacy of supervised learning in hearing aids heavily relies on the quality and diversity of the training data. The more representative the dataset, the more effective the algorithms become in real-world applications. As data collection methods advance, we can anticipate the emergence of even more sophisticated supervised learning models, further enhancing the capabilities of hearing aids.
Utilizing Unsupervised Learning Techniques
Unsupervised learning techniques constitute another vital element of machine learning applied in hearing aids. Unlike supervised learning, unsupervised learning does not rely on labeled datasets; instead, it focuses on uncovering patterns and structures within the data itself. This approach is particularly valuable for discovering insights that may not be immediately apparent.
In the context of hearing aids, unsupervised learning techniques can analyze user interactions and environmental variables to identify common sound patterns. For instance, algorithms can cluster users based on their preferences and behaviors, revealing trends that inform the development of personalized sound profiles. This capability allows manufacturers to create hearing aids that are more aligned with the unique needs of different user groups.
Additionally, unsupervised learning can enhance noise reduction capabilities by analyzing sound environments without predefined labels. By identifying clusters of sound data, these algorithms can effectively distinguish between speech and background noise, thereby improving audio clarity. This ability is particularly beneficial for users who frequently navigate complex auditory environments.
The potential for unsupervised learning in hearing aids is vast, as it facilitates continuous improvement based on real-world data. As algorithms evolve and become more sophisticated, they will likely uncover deeper insights into user preferences and behaviors, leading to further enhancements in hearing aid technology.
Reinforcement Learning Approaches in Hearing Aid Technology
Reinforcement learning signifies an innovative machine learning approach that has the potential to revolutionize hearing aid technology. This technique involves training algorithms through trial and error, enabling them to learn optimal strategies based on user feedback and environmental conditions.
In hearing aids, reinforcement learning can be utilized to optimize device performance in real-time. For example, algorithms can adjust settings based on user preferences, continuously learning from the outcomes of these adjustments. If a user prefers louder sound amplification in noisy environments, the algorithm can reinforce this behavior by making similar adjustments in future situations.
The adaptability of reinforcement learning renders it particularly exciting for hearing aid applications. As users engage with their devices, the algorithms can refine their strategies, becoming increasingly effective at meeting individual needs. This continuous learning process guarantees that hearing aids evolve alongside users, providing a more personalized experience over time.
Moreover, reinforcement learning can enhance user engagement by creating more intuitive and responsive devices. Users can enjoy a seamless auditory experience, secure in the knowledge that their hearing aids will automatically adjust to their preferences and surroundings. The potential impact of reinforcement learning on hearing aid technology underscores the transformative power of how hearing aids utilize machine learning to enhance user experiences.
Identifying Challenges and Limitations
Despite the remarkable advancements introduced by machine learning in hearing aids, several challenges and limitations persist. Addressing these issues is crucial for ensuring the ongoing development of effective and user-friendly devices that cater to diverse hearing needs.
Overcoming Technical Challenges
Technical challenges present a significant barrier to the widespread adoption of machine learning in hearing aids. One major concern revolves around the processing power required to execute complex algorithms in real-time. Hearing aids are compact devices with limited battery life, necessitating the development of efficient algorithms that can deliver optimal performance without excessive power consumption.
Recent advancements in miniaturization and energy-efficient technology are assisting in addressing these challenges. Manufacturers are exploring various approaches, such as optimizing algorithms to minimize computational demands and utilizing advanced hardware capable of effectively handling machine learning tasks. However, striking a balance between performance and energy consumption remains an ongoing challenge within the field.
Additionally, the variability of sound environments complicates the implementation of machine learning algorithms. Hearing aids must adapt to a broad spectrum of auditory situations, ranging from quiet rooms to noisy public spaces. Ensuring that algorithms can generalize effectively across different contexts while maintaining high performance is a complex task that necessitates ongoing research and development.
As technology continues to evolve, overcoming these technical challenges will be imperative for enhancing the functionality and user experience of machine learning-powered hearing aids.
Addressing User Adoption Barriers
User adoption barriers represent another significant challenge in integrating machine learning into hearing aids. While many individuals stand to benefit from advanced hearing aids, some may hesitate to embrace new technology due to concerns about complexity, cost, or perceived efficacy.
The complexity of modern hearing aids, with their numerous features and settings, can be overwhelming for some users. Older adults, in particular, may grapple with adapting to new technology and may feel intimidated by the prospect of navigating intricate controls and interfaces. To encourage adoption, manufacturers must prioritize user-friendly designs and provide clear guidance on how to effectively utilize these advanced features.
Cost also poses a substantial barrier to adoption. High-quality hearing aids equipped with machine learning capabilities can be expensive, rendering them inaccessible to many individuals. Manufacturers and policymakers must collaborate to explore ways to make these advanced devices more affordable, ensuring that individuals with hearing loss can access the benefits of machine learning technology.
Education and awareness play essential roles in addressing user adoption barriers. Increasing public knowledge about advancements in hearing technology, particularly the benefits of machine learning, can help dispel myths and encourage more individuals to seek assistance for their hearing needs.
Contemplating Ethical Considerations
The integration of machine learning into hearing aids raises significant ethical considerations that must be addressed to ensure responsible technology use. Key issues encompass data privacy, bias in algorithm development, and the potential for over-reliance on technology.
Data privacy stands as a paramount concern, as hearing aids collect sensitive information about users’ auditory experiences and preferences. Manufacturers must prioritize user consent and transparency, clearly communicating how data is collected, processed, and stored. Establishing robust security measures to protect user data is also vital for maintaining trust and confidence in the technology.
Bias in algorithm development represents another ethical consideration. If training datasets lack representation of diverse user populations, algorithms may perform poorly for specific groups, leading to inequitable experiences. Manufacturers must ensure that datasets used for training machine learning models are diverse and inclusive, accounting for various demographics, hearing abilities, and environmental conditions.
Finally, concerns regarding over-reliance on technology persist. While advanced hearing aids equipped with machine learning can significantly enhance auditory experiences, users may become overly dependent on these devices, neglecting other aspects of their auditory health or social interactions. Striking a balance between leveraging technology for improved communication and maintaining active engagement in social environments is essential for promoting overall well-being.
By thoughtfully addressing these ethical considerations, manufacturers can ensure that machine learning in hearing aids serves as a force for good, enhancing accessibility and quality of life for individuals with hearing loss.
Anticipating Future Trends and Innovations
The future of machine learning in hearing technology is brimming with exciting trends and innovations that promise to further enhance user experiences and improve hearing health outcomes. As advancements continue to unfold, we can anticipate transformative changes in how hearing aids operate and interact with users.
Progressing Algorithm Development
Advancements in algorithm development are crucial for the ongoing evolution of machine learning in hearing aids. As researchers delve into new methodologies and techniques, we can expect to see increasingly sophisticated algorithms capable of comprehending complex auditory environments with greater accuracy.
One significant area of focus involves developing algorithms that can learn from user behavior in real-time, adapting to individual preferences and environmental changes. By employing techniques such as deep learning and neural networks, manufacturers can create hearing aids that continuously optimize performance, ensuring users receive the best auditory experience possible.
Moreover, integrating multi-modal data sources, such as visual cues and contextual information, may lead to even more advanced algorithms. By analyzing data from various sensory inputs, hearing aids can gain a holistic understanding of the user’s environment, enabling them to make more informed adjustments and enhance communication clarity.
As algorithm development continues to progress, the potential for innovative applications in hearing technology is vast. Future hearing aids may incorporate features such as emotion recognition, allowing devices to adapt based on the user’s emotional state, leading to a more personalized auditory experience.
Integrating Hearing Aids with Emerging Technologies
The integration of hearing aids with other technologies signifies a significant trend in the future of hearing health. As the Internet of Things (IoT) expands, we can anticipate hearing aids that seamlessly connect with smartphones, smart home devices, and other tech solutions, enriching functionality and user experience.
For example, hearing aids could synchronize with smartphones to enable users to receive notifications, stream music, or make phone calls directly through their devices. This level of integration not only simplifies everyday tasks but also enhances social interactions, as users can engage with their devices more effortlessly.
Moreover, hearing aids may become integral components of smart homes, allowing users to receive alerts for doorbells, alarms, or other critical sounds through their hearing devices. This integration heightens users’ awareness of their surroundings and ensures they remain connected to their homes, even in noisy environments.
As technology continues to evolve, the potential for hearing aids to integrate with wearable health devices represents another exciting prospect. By monitoring additional health metrics, such as heart rate or activity levels, hearing aids may provide users with a more comprehensive understanding of their overall well-being, further enriching their experience.
Impacting Hearing Health Positively
The potential impact of machine learning on hearing health is profound. As hearing aids become more sophisticated, we can anticipate significant improvements in communication and social engagement for individuals with hearing loss. The ability to personalize auditory experiences and adapt to diverse environments fosters confidence and empowerment, enabling users to participate more fully in social interactions.
Furthermore, as machine learning technology continues to advance, we may observe increased accessibility for individuals with varying levels of hearing loss. By creating devices that are more responsive to individual preferences, manufacturers can ensure that hearing aids cater to a broader range of user needs, ultimately improving the quality of life for many.
Additionally, the integration of machine learning in hearing aids may encourage proactive approaches to hearing health. By continuously monitoring user interactions and providing insights into listening habits, these devices can prompt individuals to seek support when necessary, potentially leading to earlier interventions and better outcomes.
As machine learning continues to shape the future of hearing technology, the potential for positive impact on hearing health is immense, underscoring the importance of ongoing innovation in this field.
Innovations in Materials and Design
Emerging materials and design innovations stand central to the future of hearing aids, especially as users seek devices that are not only functional but also comfortable and visually appealing. Manufacturers are increasingly exploring new materials that enhance the durability, comfort, and overall user experience of hearing aids.
For instance, advancements in flexible and lightweight materials can lead to more comfortable designs that fit snugly in the ear without causing discomfort. Innovations such as 3D printing are also enabling the creation of custom-fitted hearing aids tailored to individual ear shapes, improving both comfort and acoustic performance.
Furthermore, design innovations that prioritize discretion and style are gaining traction among users. Hearing aids that seamlessly blend with users’ personal aesthetics can foster greater adoption and usage. By prioritizing user preferences in design, manufacturers can create devices that not only perform optimally but also resonate with users’ lifestyles and self-image.
As technology continues to evolve, we can expect to see exciting developments in hearing aid design, including the integration of smart features and user-friendly interfaces. By combining advanced technology with thoughtful design, manufacturers can craft hearing aids that enhance both functionality and user satisfaction.
Artificial Intelligence and Machine Learning Synergy
Artificial intelligence (AI) and machine learning are poised to play an increasingly significant role in customizing hearing aid settings and enhancing user experience. As these technologies continue to advance, we can anticipate the emergence of even more sophisticated algorithms that improve the functionality and adaptability of hearing aids.
AI-driven systems may enable hearing aids to analyze user behavior on a deeper level, predicting preferences and making proactive adjustments to settings. This level of personalization could result in hearing aids that not only respond to immediate user needs but also anticipate future requirements based on historical data.
Moreover, the integration of AI could enhance collaboration between hearing aids and audiologists. By leveraging AI algorithms, audiologists can gain valuable insights into user behavior, enabling them to offer more targeted recommendations and support. This partnership between technology and professional expertise can lead to improved user satisfaction and outcomes.
As AI and machine learning continue to shape the future of hearing technology, the potential for innovation remains boundless. By harnessing the power of these technologies, manufacturers can create hearing aids that truly meet the diverse needs of users, enhancing communication and enriching lives.
Frequently Asked Questions about Machine Learning in Hearing Aids
What is machine learning in hearing aids?
Machine learning in hearing aids refers to the application of algorithms that learn from data to enhance sound processing, personalize settings, and adapt to user needs in real-time.
How does machine learning improve sound quality in hearing aids?
Machine learning enhances sound quality by intelligently distinguishing between speech and background noise, facilitating clearer communication and improved listening experiences for users.
Can hearing aids automatically adjust to different environments?
Yes, hearing aids equipped with machine learning possess the capability to automatically adjust their settings based on the user’s environment, optimizing sound quality for various situations.
What are the advantages of personalized sound profiles in hearing aids?
Personalized sound profiles enhance user satisfaction by tailoring audio output to individual preferences and hearing patterns, ensuring optimal listening experiences across different settings.
How do hearing aids ensure user data privacy?
Manufacturers prioritize user data privacy by implementing robust security measures, securing user consent, and providing transparency regarding data collection and usage policies.
What challenges do manufacturers face in developing machine learning hearing aids?
Manufacturers encounter technical challenges related to processing power and battery life, as well as user adoption barriers stemming from complexity and cost considerations.
What role does user feedback play in improving hearing aids?
User feedback is essential for refining hearing aid performance, as it informs algorithms about preferences and experiences, leading to continuous enhancements and personalization of devices.
What innovations can we expect in the future of hearing aids?
Future hearing aids may incorporate advanced algorithms, seamless integration with other technologies, and improved design innovations that prioritize comfort and aesthetics for users.
How can machine learning contribute to hearing health?
Machine learning can enhance hearing health by providing personalized experiences, encouraging proactive interventions, and fostering greater social engagement for individuals with hearing loss.
What is the importance of context-aware adjustments in hearing aids?
Context-aware adjustments enable hearing aids to automatically modify settings based on the user’s environment, ensuring an optimal auditory experience tailored to real-time conditions.
Explore our world on X!
The post Hearing Aids and Machine Learning: Improving Sound Quality appeared first on The Microsuction Ear Wax Removal Network.